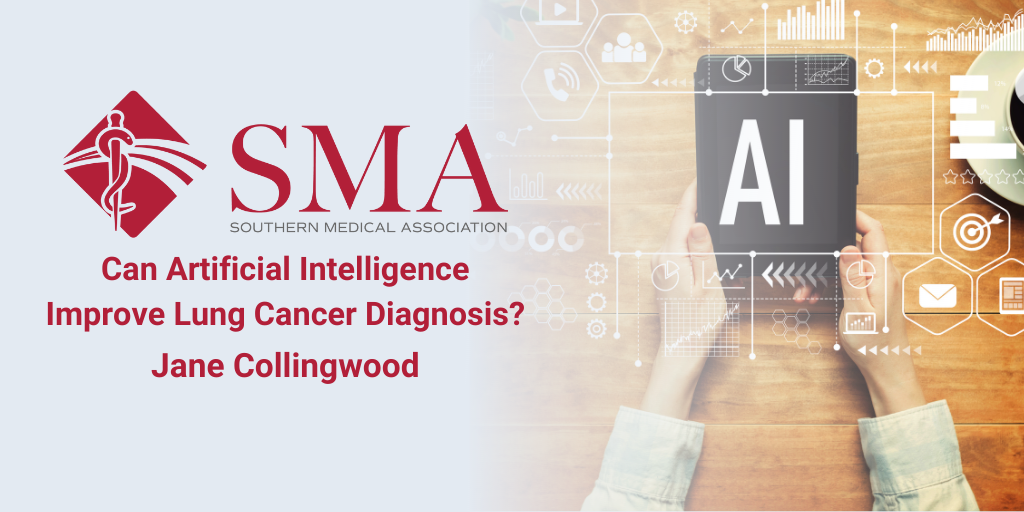
Can Artificial Intelligence Improve Lung Cancer Diagnosis?
Scientists are finding promising results using an artificial intelligence (AI) programme to identify signs of lung cancer on scans.
Although significant advances have been made in the diagnosis and treatment of lung cancer, it is still associated with poor clinical outcomes with survival strongly linked to the stage of disease at diagnosis.
The five-year survival rate for patients with early-stage disease is 56%, whereas for advanced lung cancer this is below 5%. Currently, only about 16% of lung cancers are diagnosed at an early stage, so improved screening methods are needed. Of the many screening approaches, computerised tomography is the most widely used, following a basic chest x-ray.
Nevertheless, there remains a high rate of false-positives - and procedures to confirm or rule out false-positives - so the use of artificial intelligence (AI) as a new tool for diagnosis has received much attention.
In a recent review article, Dr J. Luis Espinoza of Kanazawa University, Japan, writes that for lung cancer diagnosis, "coupling AI algorithms with available clinical and biomedical data has the potential to improve lung cancer screening methods.
"For example, AI has the potential to improve the analysis and interpretation of lung images obtained by magnetic resonance images (MRI) or computer tomography (CT) scans, and could be helpful to better decipher the clinical significance of data derived from tissue or fluid biomarkers, electronic medical record, and metagenomic data leading to improved diagnosis of lung diseases."
Dr Espinoza adds, "In the last decade, several AI models aimed to improve lung cancer detection have been reported. Some algorithms performed equal or even outperformed experienced radiologists in distinguishing benign from malignant lung nodules and some of those models improved diagnostic accuracy and decreased the false-positive rate."
Taking advantage of the unlimited capacity of computers for analysing data and images, several studies have used AI tools, including machine learning, deep learning, and others, to test whether their algorithms are capable of identifying imaging features from CT images that may be specific to lung cancer, to more accurately differentiate between benign and cancerous nodules, in order to help improve lung cancer screening.
One such study was carried out earlier in 2021 by Dr Benoit Audelan and colleagues at the University of Cote d'Azur, France. The team investigated whether a method involving AI could save time and even be more accurate than a radiologist at examining CT images for lung nodules.
They created an AI programme based on deep learning, which was trained using 888 CT scans from the National Lung Screening Trial. This was a US-based clinical trial, sponsored by the National Cancer Institute, which recruited participants between 2002 and 2004. It was conducted by the American College of Radiology Imaging Network and the Lung Screening Study Group.
The scans were also examined by radiologists. To confirm the reliability of the programme, it was then tested on 1,179 further scans.
The program was 97% effective in detecting malignant tumours in those CT scans, up to a year before the patient was diagnosed. But it also had a relatively high rate of false positives, which the authors say needs addressing before it could be rolled out.
Presenting the work at the European Respiratory Society virtual International Congress, the researchers explained that this new tool "showed good performance at detecting malignant biopsy-confirmed lesions even one year prior to their detection by radiologists".
Dr Audelan said, “Screening for lung cancer means many more CT scans being taken and we do not have enough radiologists to review them all. That’s why we need to develop computer programs that can help. Our study shows that this program can find possible signs of lung cancer up to a year earlier.
“The objective of our research is not to replace radiologists but to assist them by giving them a tool that can spot the earliest signs of lung cancer.”
Professor Joanna Chorostowska-Wynimko of the European Respiratory Society commented, “This work is promising, however, before it can be used, researchers will need to make it better at distinguishing between lung tissue that is abnormal but benign and tissue that is probably cancer.”
A further promising study was carried out by researchers from Massachusetts General Hospital, Boston, MA, and Seoul National University in Korea. This study also used information from the National Lung Screening Trial, to assess if a deep learning-based AI algorithm improves lung cancer detection on chest x-rays.
A total of 173 images were analysed, from participants with and without lung cancer. The images were assessed by three radiology residents and five board-certified radiologists.
Results showed that, with the addition of AI findings, the detection of lung cancer increased for residents, but was similar for radiologists, compared to without AI. False-positives were similar for residents, but decreased for radiologists, with the addition of AI.
"An AI algorithm can enhance the performance of readers for the detection of lung cancers on chest radiographs when used as a second reader," the researchers report. "With AI, radiology residents were able to recommend more chest CT examinations for patients with visible lung cancer," they add.
Chest x-ray, used in this study, has been shown to be unreliable as a tool for lung cancer screening, however, it is cheaper and more widely accessible than CT scanning. Hence many clinicians still consider that chest x-rays may have some utility in early lung cancer detection. The inclusion of AI algorithms has the potential to improve their diagnostic precision.
Dr Espinoza believes it is now evident that AI "could emerge as a formidable tool to refine the diagnosis of lung cancer". He points out that with the rapid expansion of computing speed and the increased efficiency of AI algorithms, it is likely that whole-body imaging data could be evaluated, rather than the current segmentation analysis.
This implies that AI algorithms could be applicable in several aspects of early lung cancer diagnosis, he explains, "including the development of risk prediction tools for the accurate identification of high-risk individuals, the precise discrimination between malignant and nonmalignant nodules, and the integration of data from imaging studies with information derived from serological and tissue biomarkers studies".
By integrating several forms of patient data, AI algorithms have a clear potential to improve the early diagnosis of lung cancer. This has now been demonstrated in several settings. Furthermore, it is likely that in future, the usefulness of AI in the early diagnosis of lung cancer may extend beyond lung images to blood samples - containing circulating autoantibodies, microRNAs, and various serum biomarkers which may highlight the presence of lung cancer.
This approach could be extended further, Dr Espinoza suggests, with "high-throughput technologies such as metabolomics, transcriptomics, epigenomics". He believes that, "The integration of multiple 'omics' together with information from medical images and clinical data will provide insightful information for the understanding of human diseases, including lung cancer."
Dr Christopher Mathew of East Sussex Healthcare NHS Trust, UK, agrees with this sense of optimism. In a recent review he writes, "Combining the performance of artificial intelligence with a radiologist's expertise offers a successful outcome with higher accuracy. Thus, we can conclude that higher sensitivity, specificity, and accuracy of lung cancer screening and classification of nodules is possible through the integration of artificial intelligence and radiology."
However, he adds, "The validation of models and further research is to be carried out to determine the feasibility of this integration."
References and Resources
- Abstract no: OA4317 “Validation of lung nodule detection a year before diagnosis in NLST dataset based on a deep learning system”, by Benoît Audelan et al, presented on Wednesday 8 September 2021 at the European Respiratory Society virtual International Congress. https://k4.ersnet.org/prod/v2/Front/Program/Session?e=262&session=13699
- Yoo, H. et al. Validation of a Deep Learning Algorithm for the Detection of Malignant Pulmonary Nodules in Chest Radiographs. JAMA Network Open, September 2020 doi: 10.1001/jamanetworkopen.2020.17135 https://www.ncbi.nlm.nih.gov/pmc/articles/PMC7516603/
- Yoo, H. et al. AI-based improvement in lung cancer detection on chest radiographs: results of a multi-reader study in NLST dataset. European Radiology, 4 June 2021 doi: 10.1007/s00330-021-08074-7 https://pubmed.ncbi.nlm.nih.gov/34089072/
- Espinoza, J. L. et al. Artificial Intelligence Tools for Refining Lung Cancer Screening. Journal of Clinical Medicine, November 2020 doi: 10.3390/jcm9123860 https://www.ncbi.nlm.nih.gov/pmc/articles/PMC7760157/
- Adams, S. J. et al. Artificial Intelligence Solutions for Analysis of X-ray Images. Canadian Association of Radiologists Journal, February 2021 doi: 10.1177/0846537120941671 https://pubmed.ncbi.nlm.nih.gov/32757950/
- Mathew, C. J. et al. Artificial Intelligence and its future potential in lung cancer screening. EXCLI Journal, December 2020 doi: 10.17179/excli2020-3095 https://www.ncbi.nlm.nih.gov/pmc/articles/PMC7783473/
About the Author:
Jane Collingwood is a medical journalist with 17 years experience reporting on all areas of medical research for online and print publications. Jane has also worked on a range of medical studies funded by the UK National Health Service within the University of Bristol in the South West of England. Jane has an academic background in psychology and has authored books on stress management and respiratory infections. Currently she is combining journalism with a national coordinating role on the UK's largest surgical research trial.